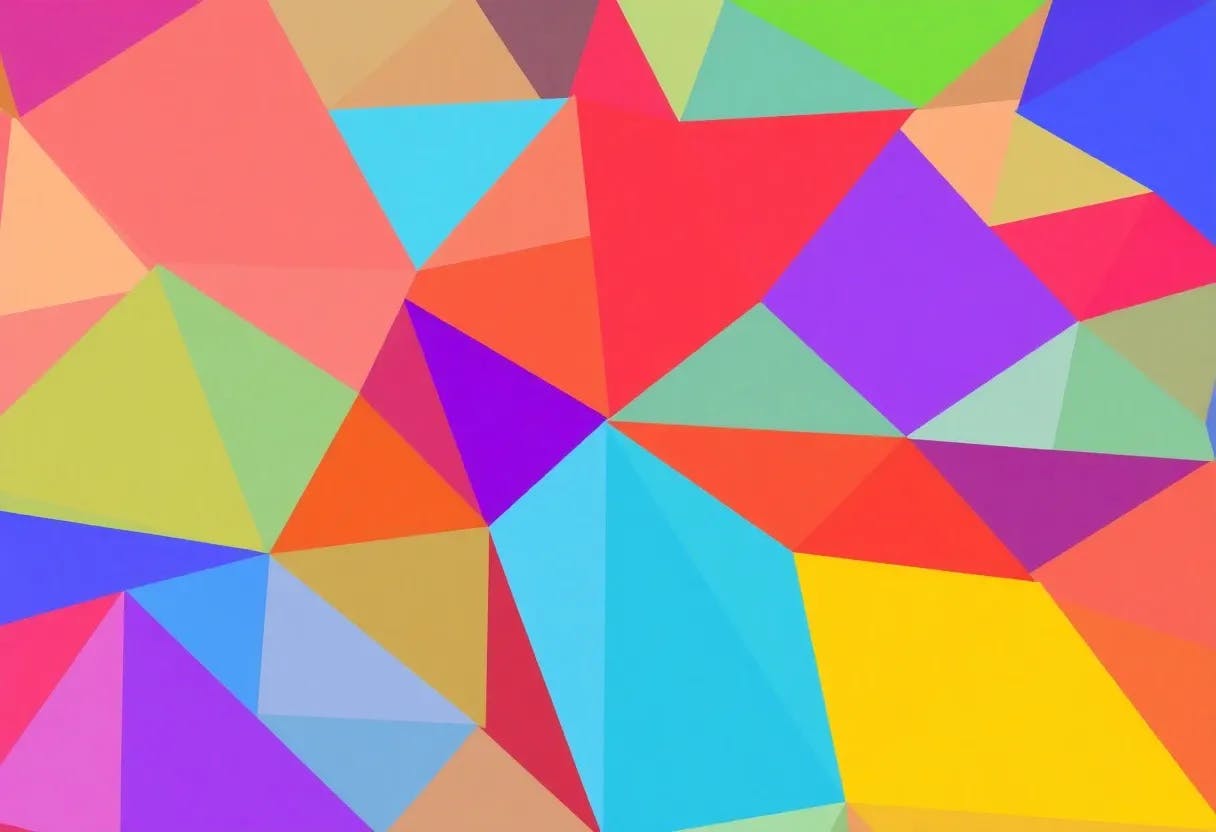
Wonder3D: Evaluating The Quality of The Reconstructed Geometry of Different Methods
3 Jan 2025
We evaluate the quality of the reconstructed geometry of different methods. The quantitative results are summarized in Table 1.
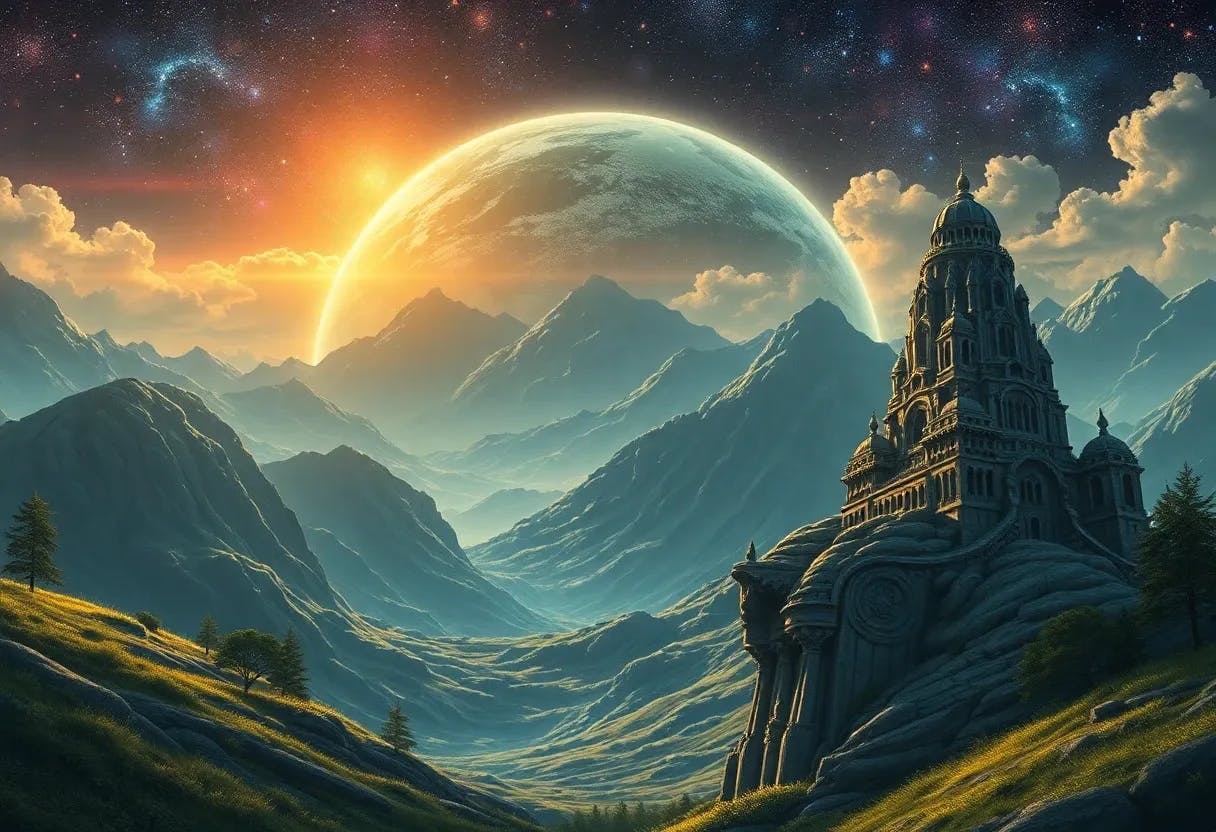
The Conclusion to Wonder3D: Future Works and References
3 Jan 2025
In this paper, we present Wonder3D, an innovative approach designed for efficiently generating high10 fidelity textured meshes from single-view images.
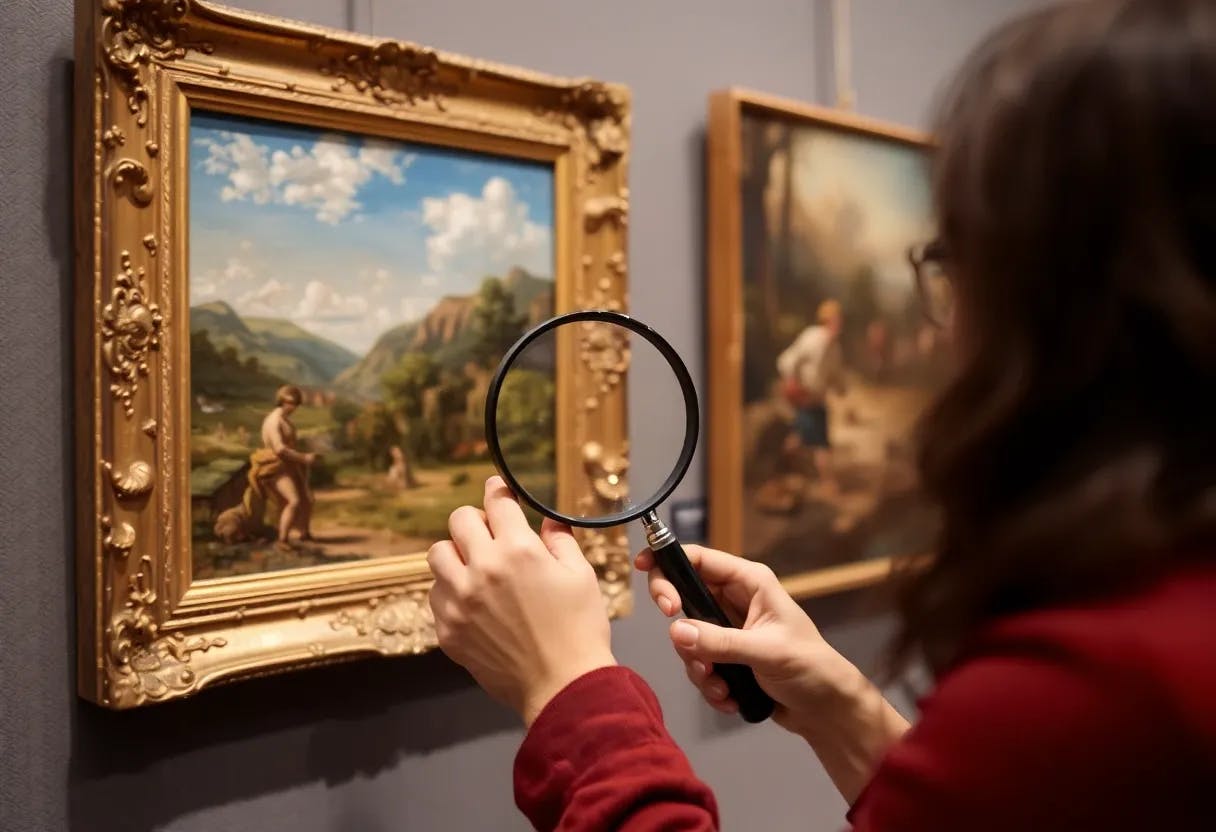
Wonder3D: Evaluating the Quality of Novel View Synthesis for Different Methods
3 Jan 2025
In this section, we conduct a set of studies to verify the effectiveness of our designs as well as the properties of the method.
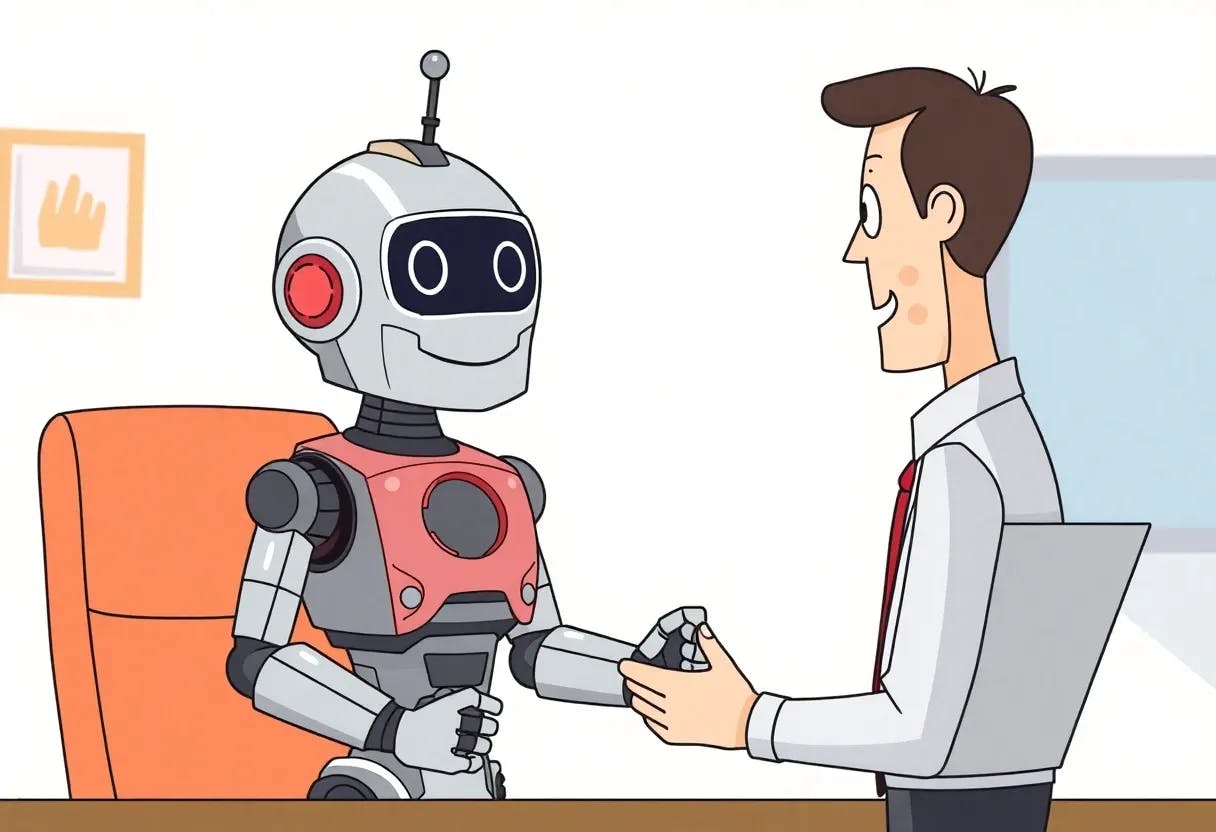
Wonder3D's Evaluation Protocol: Datasets and Metrics
2 Jan 2025
To evaluate the quality of the single-view reconstructions, we adopt two commonly used metrics Chamfer Distances (CD) and Volume IoU between ground-truth shapes
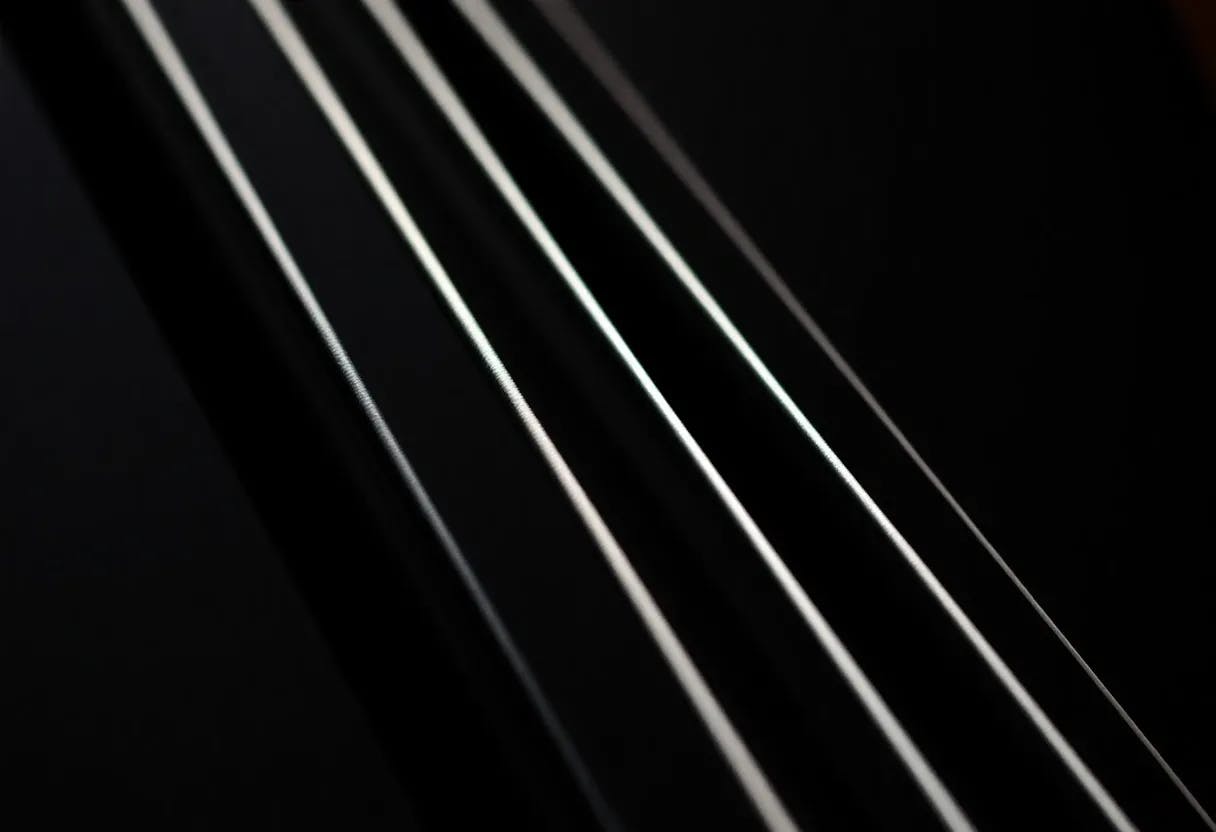
The Baseline Methods of Wonder3D and What They Mean
2 Jan 2025
We adopt Zero123 [31], RealFusion [38], Magic123 [44], One-2-3-45 [30], Point-E [41], Shap-E [25] and a recent work SyncDreamer [33] as baseline methods.
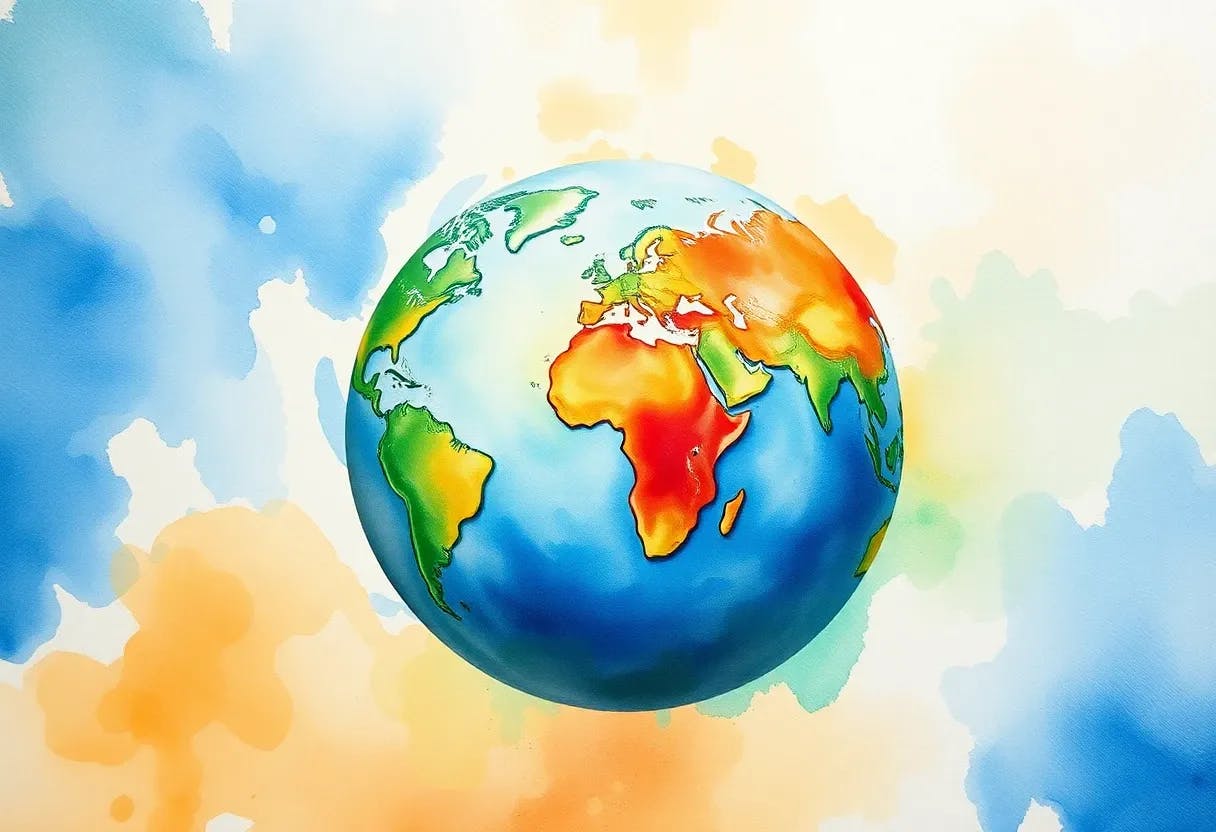
Implementation Details of Wonder3D That You Should Know About
2 Jan 2025
We train our model on the LVIS subset of the Objaverse dataset [9], which comprises approximately 30,000+ objects following a cleanup process.
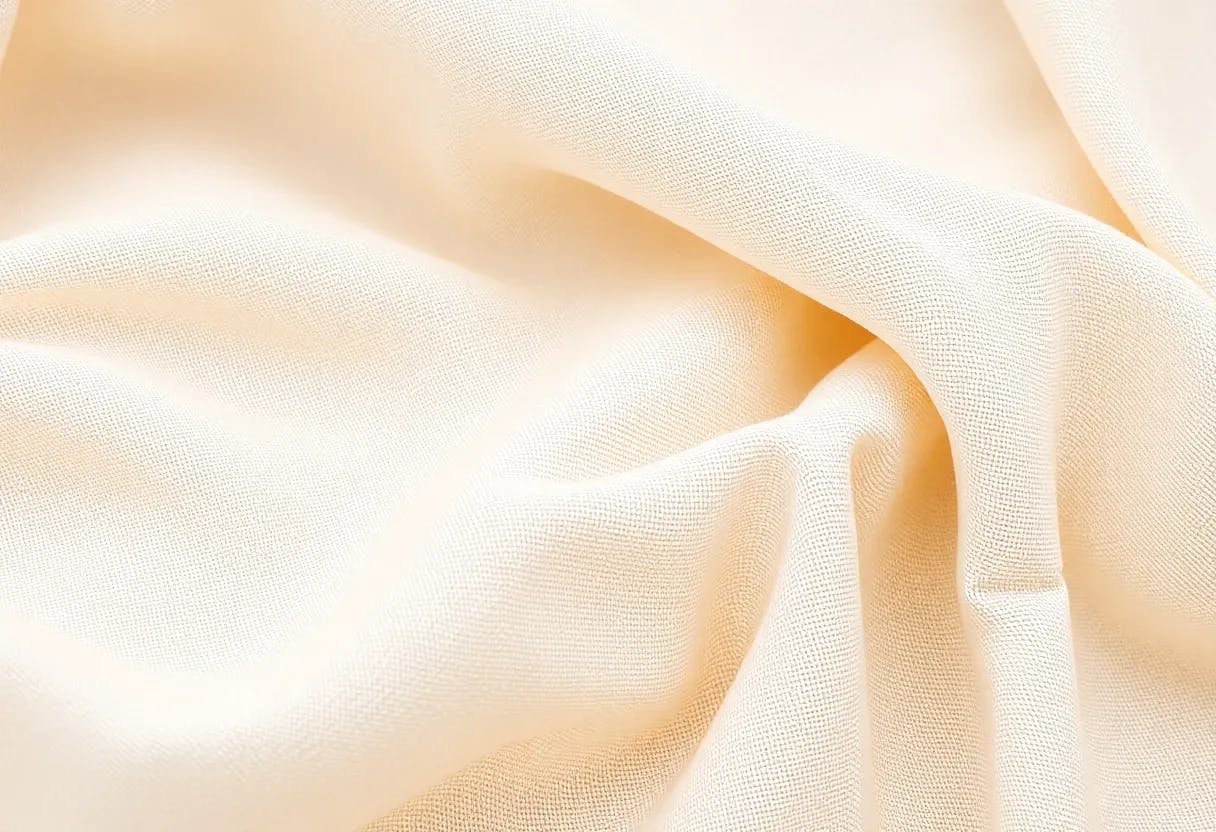
Wonder3D: Textured Mesh Extraction Explained
2 Jan 2025
To extract explicit 3D geometry from 2D normal maps and color images, we optimize a neural implicit signed distance field to amalgamate all 2D generated data.
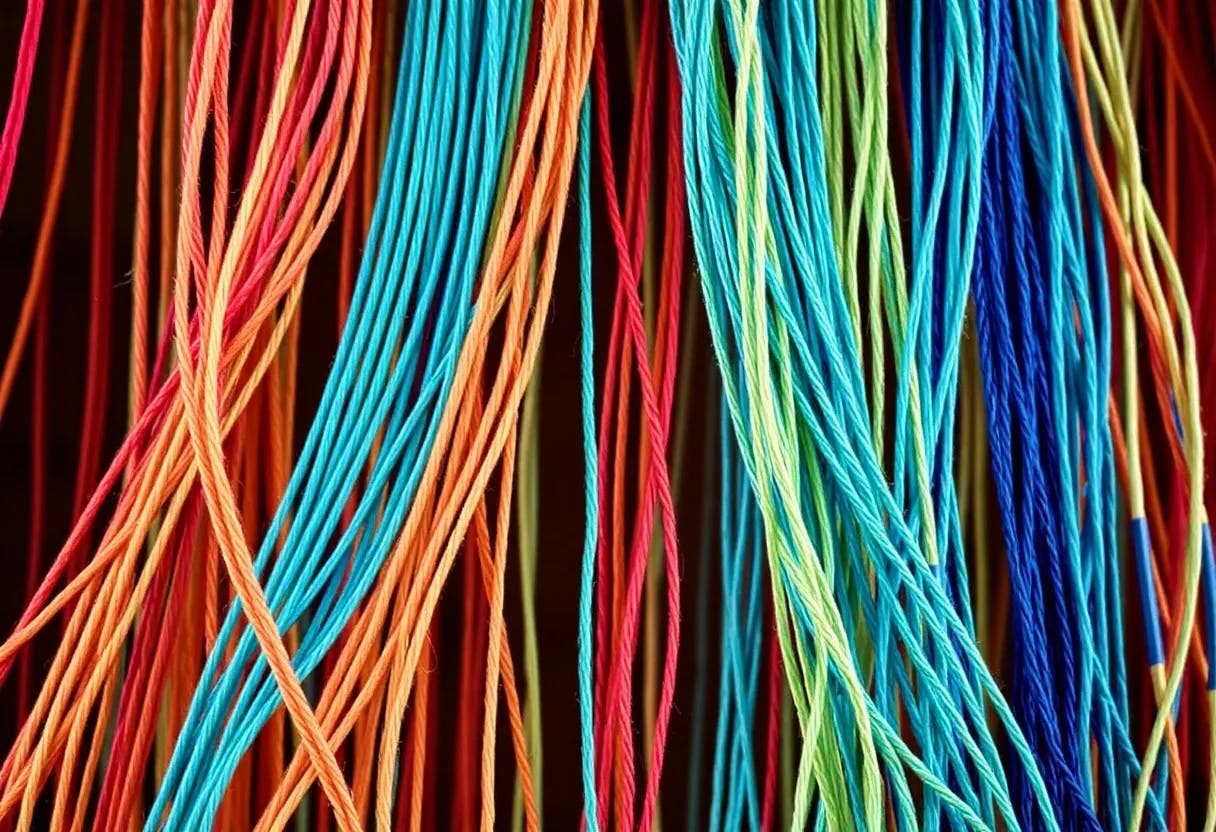
Wonder3D: What Is Cross-Domain Diffusion?
1 Jan 2025
Our model is built upon pre-trained 2D stable diffusion models [45] to leverage its strong generalization.
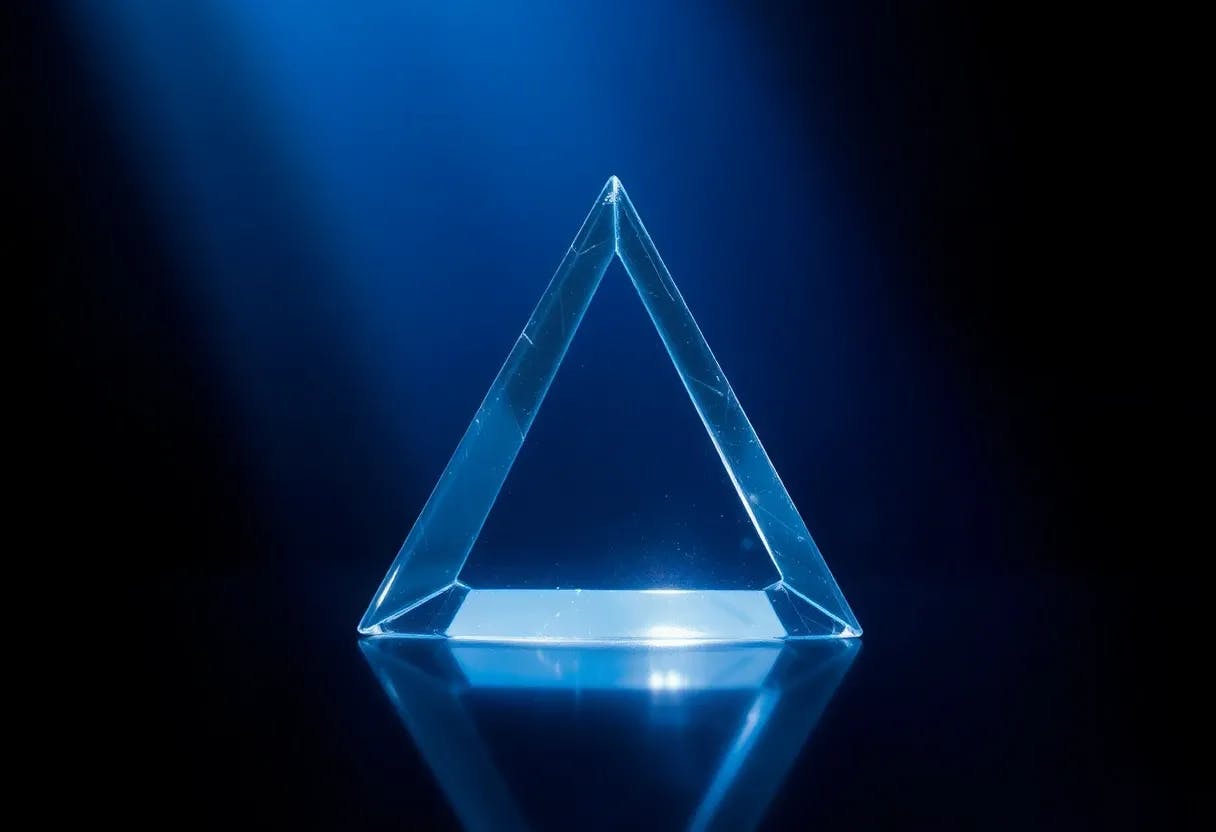
Wonder3D: A Look At Our Method and Consistent Multi-view Generation
1 Jan 2025
We propose a multi-view cross-domain diffusion scheme, which operates on two distinct domains to generate multi-view consistent normal maps and color images.